Edge Computing
Think Big. Go Small.
Neither a startup nor technology could disrupt our world quite in the same way as the pandemic currently facing humanity. “Going small” has never felt so real as we hunker down in our lives and businesses. Suddenly, the need to decentralize computing and enable more nimble processing at the source of data spans all industries.
Scientists at the University of Massachusetts, Amherst, have recently developed a portable surveillance device that can monitor spread of respiratory illnesses and flu trends using machine learning models. Their edge computing platform, FluSense¹, analyzes data on coughing sounds and crowd size in real time and could help track the spread of COVID-19. Such edge computing systems that can provide greater agility in processing real-time data, insights, and automation at the source will emerge as a big trend as we seek to build new bridges between humans and things.
By 2025, the research firm Gartner predicts 75% of all enterprise-generated data will be created and processed outside a traditional centralized data center or cloud, compared to 10% today. In our previous article, we emphasized how applications, not standards, are driving the IoT market with a multitude of use cases and a wide array of personas for any given vertical. Across today’s IoT landscape, asset and application sprawl, harsh industrial environments, limited compute per node, security vulnerabilities, and changing network conditions persist as challenges, paving the way for dedicated edge computing infrastructure to unlock real value.
1 FluSense: https://www.umass.edu/gateway/feature/flusense
By 2025, Gartner predicts 75% of all enterprise-generated data will be created and processed outside a traditional centralized data center or cloud.
Contextualizing the Edge
Think of the IoT landscape as a four-player chessboard with varying degrees of “coopetition” among the four key incumbent vendors: IT, Industrial OEM, Cloud, and Telecom, each viewing the edge computing market through a different lens.
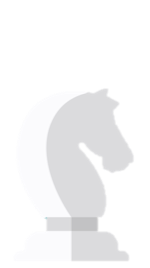
IT
Edge means customer onsite IT infrastructure, e.g. a server rack in a data center or an access point in a warehouse.
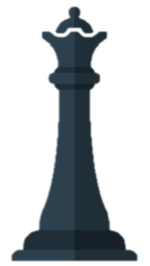
Industrial OEM
Edge compute deployments mean a video camera or a robotic arm on the factory floor or a connected fleet of vehicles.
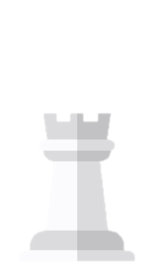
Cloud
Everything outside the core cloud is edge.
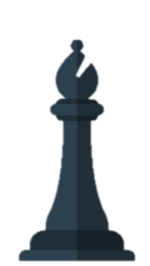
Telecom
All deployments from the cell sites to the core span the edge.
Edge Computing Investor Interest
On the Rise
According to the startup tracking platform Tracxn, VC investors funded approximately $500 million in edge computing startups from 2015 to early 2019, a modest percentage of the overall investment in IoT. However, from H2-2019 to Q1-2020, several large financings transpired, including Hailo Technologies, an Israel-based AI processor solution for edge devices, which raised $60 million in Series B funding, and edge AI-focused chip makers Kneron and Untether AI secured $40 million funding in January 2020 and $20 million funding in November 2019 respectively. Swim.AI, the data processing and edge analytics software company, raised $18.4 million in late 2019 and Vapor IO, the micro data center and edge computing platform, raised $90 million in Series C funding in January 2020. Edge computing platform Aetheros secured $15 million in Series A funding in November 2019, while edge intelligence software startup Foghorn raised $30 million in Series C funding in August 2019.
Acquisitions have also spiked over the last 12 months. For example, Equinix acquired Packet for $335 million in March 2020 with the data center and colocation company planning to leverage Packet’s technology to accelerate the development and delivery of its interconnected edge services.
Earlier this year, Apple acquired Xnor.ai in a deal expected to boost Apple’s on-device AI capabilities and competitive advantages in computer vision.
Also, Siemens acquired Pixeom in October 2019 to strengthen its Industrial Edge portfolio by adding software components for Edge runtime and device management.
The edge computing market (spanning hardware, software, and services) is expected to grow from $2.8 billion in 2019 to $9.0 billion by 2024, at a CAGR of 26.5%, according to MarketsandMarkets². With growing maturity of edge platforms and the concurrent adoption of complementary technologies such as 5G and machine learning, investments in edge computing are expected to increase.
2 MarketsandMarkets, “Edge Computing Market by Component (Hardware, Platform, and Services), Application (Smart Cities, IIoT, Content Delivery, Remote Monitoring, AR and VR), Organization Size (SMEs and Large Enterprises), Vertical, and Region - Global Forecast to 2024”
We expect edge computing technology to evolve from independent siloes to being more “cloud-like” in this decade, progressing through three cycles.
Evolution of Edge Computing
Over the Next Decade
We expect edge computing technology to evolve from independent siloes to being more “cloud-like” in this decade, progressing through three cycles.
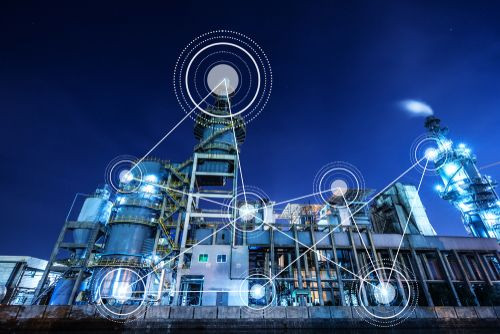
COMPUTE AT THE EDGE
For the last few decades, we’ve observed traditional light footprint computing at the edge, predominantly for capture of telemetry information. Market watchers expect the trend to continue as the industry provisions more edge computing closer to remote users and specific data sources, though future growth in adoption of this first edge iteration will likely be limited.
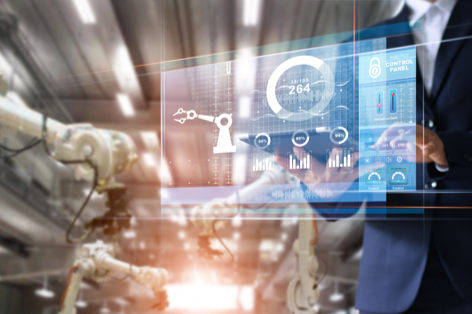
EDGE AS EXTENSION OF CORE
We have also witnessed growing adoption of ruggedized servers and IoT gateways with a software control plane spanning the core IT. By combining ruggedized hardware with a software platform, customers would be better equipped to run applications at the edge.
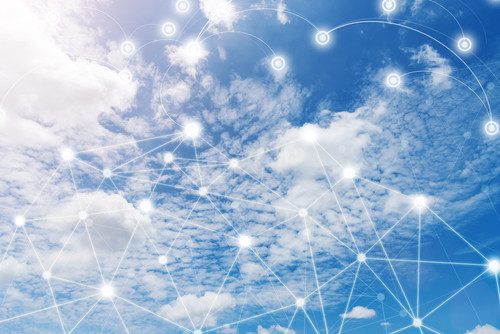
EDGE CLOUD
This is edge as part of a seamless cloud architecture. Edge cloud extends some capabilities of the cloud (including but not limited to storage, computing, network, AI, and security) to edge nodes, via a tight coupling of computing and networking hardware. Software orchestrates this fine symphony of inter-node and cloud communication, as in the case of Cisco’s Edge Intelligence software combined with Cisco’s IoT networking portfolio. The days of edge cloud are still early, and all forms of edge computing are likely to co-exist for the next couple of years.
Opportunities We See
at the Edge
Edge computing will drive a use case-dependent evolution of the tech stack – requiring new hardware form factors and software capabilities to address scale and complexity of distributed infrastructure. We believe that several opportunities exist, both on infrastructure and applications fronts.
Edge Infrastructure Opportunities
“Edge as a service” platformsColocation of massive data centers close to large-scale, distributed IoT endpoints is neither a practical solution nor does it address the networking challenges of today’s edge computing implementations. Instead, a new paradigm of software-defined networking is primed to balance the distributed nature of IoT endpoints-driven data generation while addressing the demand for low latency among edge use cases. Opportunities will proliferate for edge computing and networking platforms that enable this fine orchestration of data and workloads across various nodes with container support to run distributed applications. Startups like Pixeom, ClearBlade, and Vapor IO play in this segment.
Data Management and StreamingThe scalability, latency, and reliability demands of modern data streaming IoT applications (e.g. live video streams) running on distribution edge infrastructure are disrupting the edge cloud stack today. Controlling such a high rate of data flow and consequently a high rate of state change using the traditional, stateless data cache and streaming models has proven incredibly difficult.
Edge-native distributed databases and pub-sub platforms for real-time stateful apps will prosper with their ability to manage challenging IoT data streaming conditions, such as unreliable WAN, lossy time keeping, and lack of consensus. Acting on this thesis in 2016, Cisco Investments invested in PubNub, a data streaming network for modern, real-time IoT and communications applications. Startups like Swim.ai and Macrometa illustrate complementary edge-native database and data cache functionalities.
Edge Cloud for 5G5G and edge computing are two inherently linked technology paradigms for delivering an enriched application experience. Edge cloud supports 5G adoption with local, rather than regional, compute resources that can address 5G’s high-bandwidth, low-latency (1ms) requirements.
While network functions have traditionally run on purpose-built appliances, general-purpose edge compute and edge clouds are deploying virtualized network infrastructures for 5G to replace many dedicated hardware-based elements with virtual network functions (VNFs), especially for packet forwarding and security. The combination of network function virtualization (NFV) and edge computing makes it easier than ever to manage the lifecycle and configuration of these new VNFs and helps 5G deliver on its promise of enhanced application performance.
By localizing applications at the edge closer to end users, both network transit latency and reliability improve, driving further adoption of technologies, such as industrial robotics and drones, vehicle-to-everything (V2X) communication, AR/VR infotainment, autonomous vehicles, and associated business models.
Hardware acceleration with ML focused chipsThe advent of real-time applications in IoT leveraging machine learning has made it clear that inference needs to happen at the edge, rather than in the cloud. Software innovation is not enough to deliver the required results for such use cases with the current breed of general-purpose hardware.
Rather, we need new computing hardware that meets IoT edge characteristics, such as small form factor and low power consumption, while delivering more robust compute. We are seeing growing adoption of on-device chipsets optimized for faster, more secure, use case-specific inference developed by Intel, Google, and startups such as Mythic.ai and Untether.ai.
Edge Application OpportunitiesEdge devices collect vast amounts of information for real-time processing, which leads to the biggest opportunities for edge applications within three key categories: Sense, Automate, and Analyze.
Edge as a sensorBy 2025, the total installed base of IoT connected devices is projected to reach 75 billion worldwide³, the largest proportion of which will comprise inexpensive sensors deployed to generate and collect real-world data about ourselves, our machines, and our environment. These edge sensor networks form the lifeblood of real-time applications and offer significant opportunities for companies that are creating the foundation for edge computing through proprietary sensor-based data moats. Miovision, a McRock Capital portfolio company, is one such company empowering “smart cities” by accumulating one of the world’s largest video traffic data repositories, counting more than 9 billion vehicles and 850 million cyclists and pedestrians. Through sophisticated sensors and AI, Miovision brings visual data collection roadside to help cities sense and understand what’s happening at any intersection in real-time. Other examples of startups leveraging edge as a sensor include McRock’s portfolio company Skyspecs and Cisco Investments’ Kespry, both of which enable drone-based data collection at scale. Skyspecs uses intelligent sensors and cameras on autonomous drones to change the way inspections are carried out for onshore and offshore wind turbines, while Kespry solves the challenges of collecting survey data for industries like mining, construction, and insurance to name a few.
Smart edge with ML-driven automationRobots as well as self-driving cars and trucks feature use case-driven, full-stack applications that leverage edge computing, machine learning, and other modern technologies. For these reasons and more, we’re seeing massive value-creation opportunities with smart machines operating at the confluence of edge computing and machine learning. For example, AImotive, a Cisco Investments portfolio company, provides full-stack, AI-based autonomous driving solution for cars, including edge computing hardware for AI inference in the car and automated driving software. Almotive’s edge compute platform gives the car’s smart sensors and compute clusters real-time inference and autonomy. Even today, in the fight against COVID-19, we’re witnessing smart edge applications rise to the occasion, including the use of robots to ensure social distancing, especially in high-risk areas. Across several provinces in China, hospitals are using robots to deliver food to patients, to help clean the facilities, and to avoid unwanted contact by navigating patients between hospital zones.
Edge driving real-time analyticsUnlike traditional analytics models that depend on centralized data warehouses and lakes, edge analytics models collect, process, and analyze data at the edge with an emphasis on speed and decentralization. Over time, we expect use case and device-optimized machine learning models running at the edge to provide more value. Consider startups such as FogHorn and Litmus Automation, which have employed the ML approach to offer closed loop edge analytics solutions for industrial customers.
3 Source: Statista - Internet of Things (IoT) connected devices installed base worldwide from 2015 to 2025
Scaling
Edge Startups
Despite the potential for unlocking massive value, edge computing has yet to result in many scaled up startups or large-ticket acquisition deals to date. We have some thoughts on this.
While still in a nascent stage, the edge computing market continues to carry high customer risk aversion, namely for the extreme diversity that characterizes the different systems and networks across locations. Let’s dive deeper into specific challenges and success factors.
Edge InfrastructureInfrastructure platforms for edge, such as Edge as a Service and Edge Cloud for 5G, need to provide platform features, such as low- or no-touch remote provisioning and management, security, scalability across locations and network conditions, as well as out-of-box integrations with incumbent IT and OT solutions. Whereas startups may struggle to bring all these features at the outset and serve as the chosen platform for edge implementations, incumbent platforms in computing, network management, and automation markets are much better poised for success in this regard.
Startups offering use-case specific infrastructure components and tools will complement the broad offerings of edge platforms. One such opportunity lies in data management for tools to offer ingestion, transformation, streaming, synchronization across edge nodes and cloud, and governance features to complement the edge infrastructure platforms. Also, startups will find opportunities in providing technologies that support machine learning inference at the edge, including software optimization to run ML models on low-power, low-compute edge devices, such as Xnor.ai, and hardware acceleration with ML-focused chips.
Edge ApplicationsThe following strategies can help applications startups win at the edge:
1. Tap into existing budget with measurable ROI: Edge applications should target a broader story of pervasive connectivity, sensors, and business-critical use cases where the ROI is clear to demonstrate. As part of digital transformation or Industry 4.0 initiatives, most enterprises have existing or emerging budget allocations for AI, 5G, automation, remote monitoring, and predictive maintenance, all of which typically have an edge component.
2. Continuously learn and improve: As more data is generated and captured at the edge, new opportunities will emerge for expansion into greater use cases and those deeply entrenched within customers’ accounts.
3. Leverage the cloud: It’s not edge versus cloud; it’s edge and cloud as synergistic technologies complementing one another. Edge startups should partner with leading cloud players, wherein edge startups bring domain-specific solutions to the partnership, while cloud vendors bring top-down sales motion in addition to cloud compute and storage.
4. Optimize spend: Startups should optimize their infrastructure use, accounting for the following:
Compute and storage: Much of data collected at the edge is often disposable as it does not reflect a useful or critical change in the operation or condition being monitored. Therefore, startups should be smart about the way they sample, store and infer edge data.
Network bandwidth: Much edge-generated data is only valuable at the edge and is at times only useful for a short period of time (few hundreds of milliseconds).
5. Partner with incumbent IT and OT platforms: In a nascent market, it is tougher for startups to scale customer accounts beyond trials. Best-case scenarios are partnership models in which the startups’ application is part of a bigger project with pull-through from incumbent IT and OT platforms.
Let’s build the next-generation edge together
If you are an IoT startup and would like to partner with us to build the next-generation edge, reach out to McRock Capital and Cisco Investments. And be sure to catch the next edition of this exclusive three-part series where we will share our take on AI-driven automation in the Industrial IoT space.