Analysts suggest that 90 percent of the world’s data was generated within the past two years, but only a small percentage of that data is actually used to drive insights.
AI promises us a world in which cars can predict when to swerve, to avoid hitting mothers crossing the street with their babies. It promises us refrigerators that can predict what food to order before you run out. It promises us networks that can predict when to heal themselves before they become overloaded. AI promises us a world, underpinned by predictions based on data, in which our machines will know what we want and need before we ourselves do.
In order to realize that vision, we need to address the very real hurdles that stand in the way of operationalizing predictive analytics, which are empowered by Machine Learning (ML). ML is software that learns by example, ingesting data and tuning algorithms to predict likely outcomes, using a variety of use-case specific input variables For example, if you’ve ordered eggs at 6 p.m., every Thursday over the past 12 months, ML will tell us that you’ll likely want to order eggs again next Thursday, at 6 p.m.
Why don’t we use more data to drive better decisions? We face a major problem today in that machines and software scale well, but humans do not. Our data has grown at a much faster pace than our population of data scientists, who represent a relatively small and high-demand subset of today’s workforce. According to a recent 451 Research survey, 36 percent of companies cited lack of skilled workers as the most significant barrier to deploying machine learning.(1)
Why is ML limited largely to skilled workers?
Machine learning is operationally hard. Data science projects in general require a lot of manual input. In spite of great strides towards easier data management, data today is still relatively messy (disorganized, incomplete, error prone), slow (batch-oriented, vs. real-time), and heavy (difficult to move).
If you were to launch a machine learning project today, you’d probably first collect data from a variety of data sources (databases with different organizational schemes, data from legacy systems, etc.). Then you’d likely clean that data as a next step (correct for incompleteness, errors, match variables that may comprise the same information but that are labeled differently – e.g., “phone number” vs. “mobile #”). As your third step, you might choose and apply a learning algorithm to your data, in order to ultimately produce a predictive model for whatever you’re hoping to forecast. The final step is to deploy the model and then monitor it so when accuracy degrades over time (typically as the business changes), the model can be refreshed and redeployed.
On your first try, you might end up with a predictive model that works.
Or, you might end up with a predictive model that fails to offer a representative view of the relationship between your input variables and predictive output.
Or, you might end up with a predictive model that perfectly fits the data to your sample, historic data, but fails in the real world, with a larger and more current data set.
So what would you do? You could go back to your data and increase or decrease the number of input variables. You could choose a different learning algorithm to generate your predictive model. You could choose a different set of data from a different set of sources. You could change your threshold of acceptable accuracy for your model (maybe acceptable for when your refrigerator needs to order eggs for you, but probably not acceptable for your car to decide when not to hit someone).
Bottom line, ML is a difficult and particularly iterative problem to solve.
How could we empower less technical users to put ML into practice?
We first saw DataRobot in action at the Strata Data Conference in New York in 2017. One of their sales people demoed the product, explaining that a key goal of the company was to put the power of machine learning into the hands of business analysts.
The first thing we noticed looking at DataRobot’s interface was the giant “Start” button in the middle of the screen. DataRobot, as their sales person explained, aimed to do for machine learning what the point and shoot camera did for photography – simplify a complex technical process into the shortest number of steps.
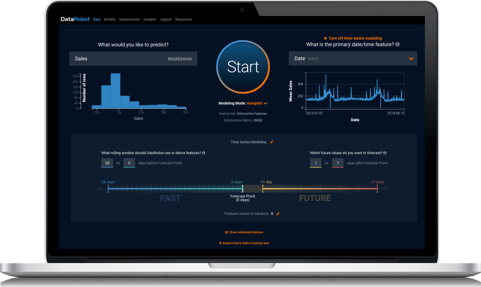
DataRobot’s sales person also explained that their ultimate goal was to create a clear link between machine learning and ROI impact. That is, they aimed to help the business analysts, versus the data scientist, understand the link between predictive analytics and business problems.
Over the next year, we invested time into better understanding the ML landscape. We met with dozens of companies operating in the space – some that sought to take more of a DIY approach towards the ML problem, some that specialized in simplifying certain parts of the ML project lifecycle, and some that were oriented towards solving specific ML use cases (i.e., applying ML to specific industry verticals).
At Cisco Investments, one of our greatest assets in our diligence process is our access to the Cisco community. We interviewed a number of our data scientists who gave DataRobot a spin.
Typical feedback from our data scientists: “a normal ML project could take us days, if not weeks, and DataRobot’s product allows us to streamline that process into hours, while still allowing us to ‘get under the hood’ and tweak what we need to tweak, in order to make our models work in the way we want them to.”
We also interviewed business analysts, and sought to validate the thesis that machine learning could be applied by less technical users towards problems that would typically have fallen under the purview of broad-based business intelligence, but with a predictive lens.
We found that business analysts were using DataRobot to address a wide range of problems: for example, predicting credit card fraud before a purchase is complete, predicting how to best staff call centers, before customers call to complain, predicting when to replace hardware, before it breaks.
We saw clear potential applications for DataRobot’s technology, both for our own purposes, and for our customers’ purposes.
Over a long courting period, we learned more about DataRobot’s team and company philosophy. DataRobot’s DNA is in the machine learning space – the company was founded by former data scientists from Travellers Insurance who were top performers on Kaggle, a platform for predictive modeling and analytics competitions. Its growing team has deep roots in the broader enterprise software space, and in the markets it serves. The company as a whole offers an impressive level of focus on its mission – a category creator pioneering machine learning and linking ML as a practice to ROI as a result.
We invested in DataRobot’s recent fundraising round, with the belief that ML offers massive potential to Cisco, its customers, and its partners, and with the belief that DataRobot offers one of the best platforms in the market today to empower more people than ever to make use of ML.
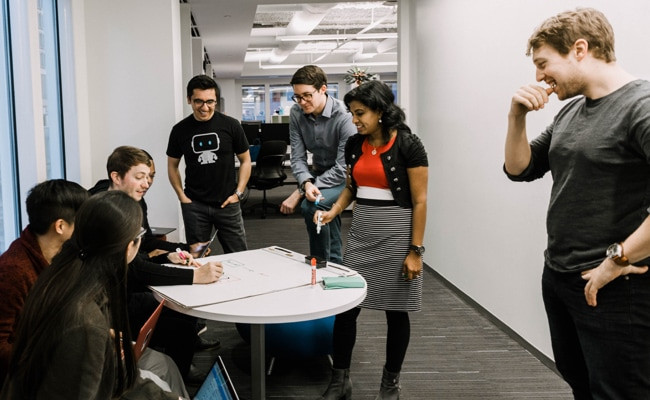
What’s next?
We take a thematic approach towards investing. We identify key market trends, and seek to partner with the best companies in the market that capitalize on those trends. With respect to data and analytics, a few overarching themes dominate today’s headlines:
Proliferation of Data - driven by sensors, machine data, and new architectures
Shift to the Cloud – rapidly changing dynamics of data gravity
Passive to Active – moving from historic analysis, towards active remediation
We see a future where a broad set of users, from the non-technical business analyst to the data scientist to the engineer, will leverage massive data sets from inside and outside of their organizations to solve problems before they occur.
In order to realize this vision of operationalizing predictive analytics, we are looking to the startup ecosystem to offer tools that catalog and store information more elegantly, tools that offer access to data almost instantly, and tools that automatically solve problems identified through data-driven insight.
Sources:
(1) Skills gap is the biggest barrier to enterprise adoption of AI and machine learning, 451Research, September 2018.